Jun 02, 2023
discover the top ai assistant of 2023
AI assistants, particularly the AI Assistant, have become an integral part of our lives, transforming the way we interact with technology and providing us with unprecedented levels of assistance in various aspects of life. This blog post aims to compare and contrast the top AI assistants of 2023, including the AI Assistant, helping you make an informed decision on which one is right for you.,- Discover the top AI assistant of 2023, including personal, voice, writing, scheduling and productivity assistants.,- Compare factors such as features, pricing and user experience to make an informed decision when selecting the right AI assistant for your needs.,- Anticipate more humanistic & personalized AI assistants in 2025 with enhanced capabilities to maximize productivity & organization., ,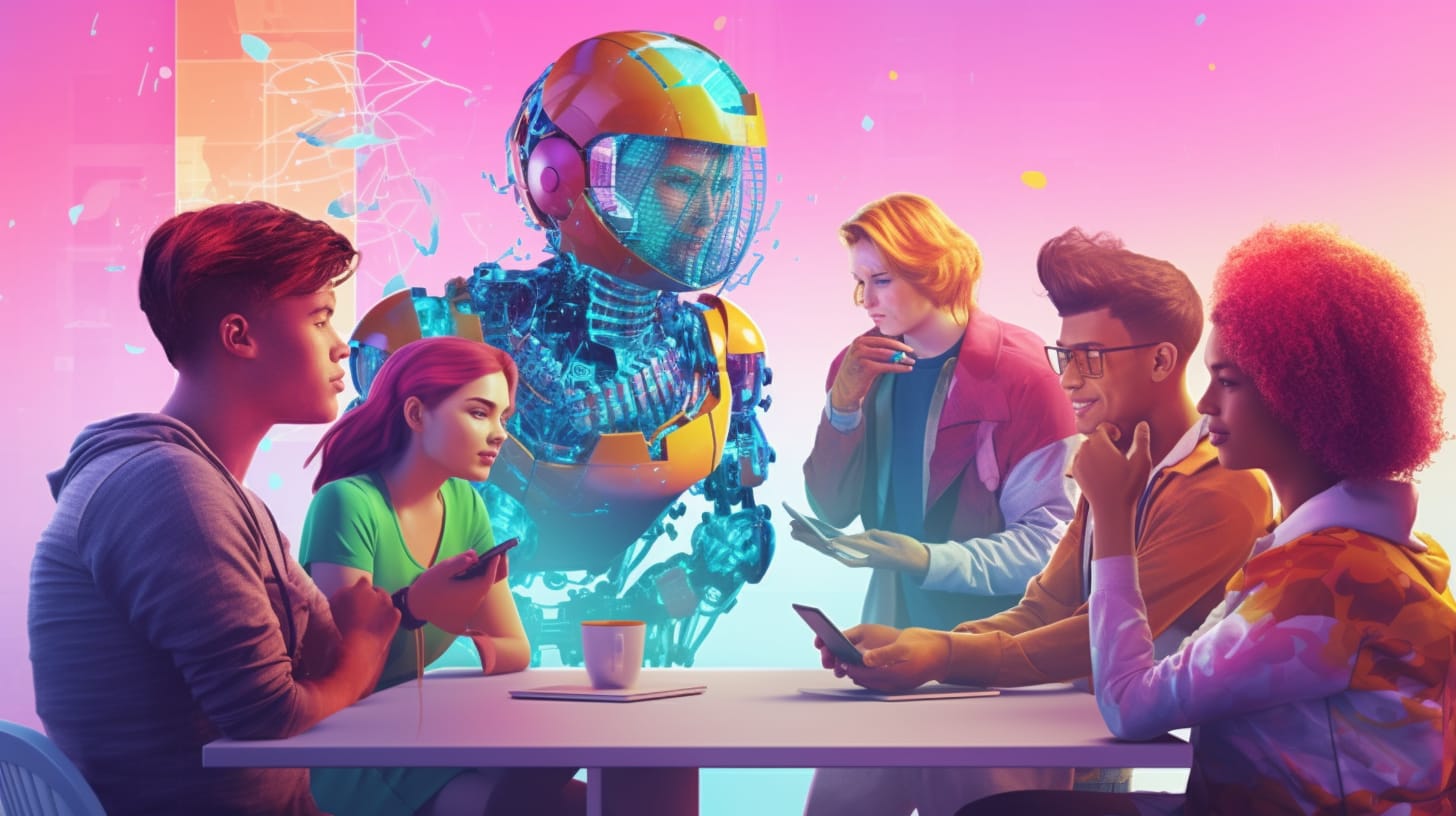,AI assistants are virtual programs designed to understand natural language commands and perform tasks, such as scheduling phone calls and send or taking dictation and other expectations from artificial intelligence ai assistants. They have the potential to save us time and effort by automating various tasks, ranging in benefits from setting reminders and looking up phone numbers to placing phone calls and reminding us about appointments. These virtual artificial intelligence ai assistants, can be found on various platforms such as smartphones, smart speakers, instant messaging apps, and AI chatbots that employ generative AI.,With the rapid advancements in AI technology, there are a large number of AI assistants on the market, each with its unique features and benefits. Some popular examples of 10 best ai and 6 ai virtual assistants and the best ai voice assistants include Google Assistant, Siri, and Amazon Alexa. It is essential to set realistic expectations from your expectations for what the best ai assistants may have transformed, and the best ai assistants may have are often being made, for what AI assistants can do and understand their capabilities to make the most of them.,There are several types of AI assistants that cater to different needs, including personal, voice, writing, scheduling, and productivity assistants.,In the following sections, we will delve into each of these categories, discussing their features and popular examples.,Personal AI assistants are AI-powered virtual assistants that can assist with tasks, including scheduling, productivity, and customer service. Some popular examples of personal AI assistants include Conversica, Kore.AI, DataBot, Hyro, and Moveworks, each offering unique features to enhance productivity and performance. Conversica, for instance, ai powered virtual assistant, boasts two-way dialogue in real-time and utilizes natural language processing, deep learning, and business process automation to boost the productivity of working team members.,Kore.AI offers tools for designing, testing, training, deploying, analyzing, and controlling intelligent virtual assistants, as well as a selection of over 35 voices and more than 100 languages for customer contact. DataBot, on the other hand, is available on multiple platforms, including Android, iPad, and Windows 10, and utilizes Google searches, Wikipedia, and RSS channels to provide answers to inquiries.,These personal AI assistants provide realistic expectations from ai wide range realistic expectations from ai amount of functionalities, tools offer many features, catering to diverse user needs and preferences.,Voice AI assistants are AI-driven virtual assistants that utilize voice recognition technology to respond to vocal commands. These assistants are available on many devices and offer various services, such as voice commands, voice searching, voice-activated device control, task handling, online information search, and real-time translation. Popular examples ai voice assistants include Google Assistant, Siri, and Amazon Alexa.,Google Assistant is one of the most advanced artificial intelligence voice assistants, offering a wide range of functionalities like setting alarms, playing audiobooks, streaming podcasts, and providing up-to-date information on news, weather, and sports. Siri, Apple's voice AI assistant, is another popular choice among users, providing similar features and capabilities.,These voice AI assistants have become increasingly sophisticated, offering users a seamless and convenient way to interact with technology.,Writing AI assistants are software tools that use artificial intelligence to help users produce high-quality, original content in a much shorter duration of time than manual writing alone would require. They employ natural language processing (NLP) and machine learning algorithms to analyze user input and produce content tailored to the user's requirements. These AI assistants can expedite the process of producing high-quality content and assist in creating content that is captivating and tailored to the intended audience.,One popular writing AI assistant is the 10Web AI Writing Assistant, which helps users optimize their content SEO by rectifying issues directly in Yoast, generating or rephrasing meta descriptions and titles, and correcting paragraph/sentence length errors to optimize your content for seo and readability errors. Another example is ELSA Speak, an AI assistant that helps users improve their English speaking skills. Ai writing assistants have become a valuable tool for content creators, reducing the time and effort required to produce high-quality content and avoid seo and readability errors.,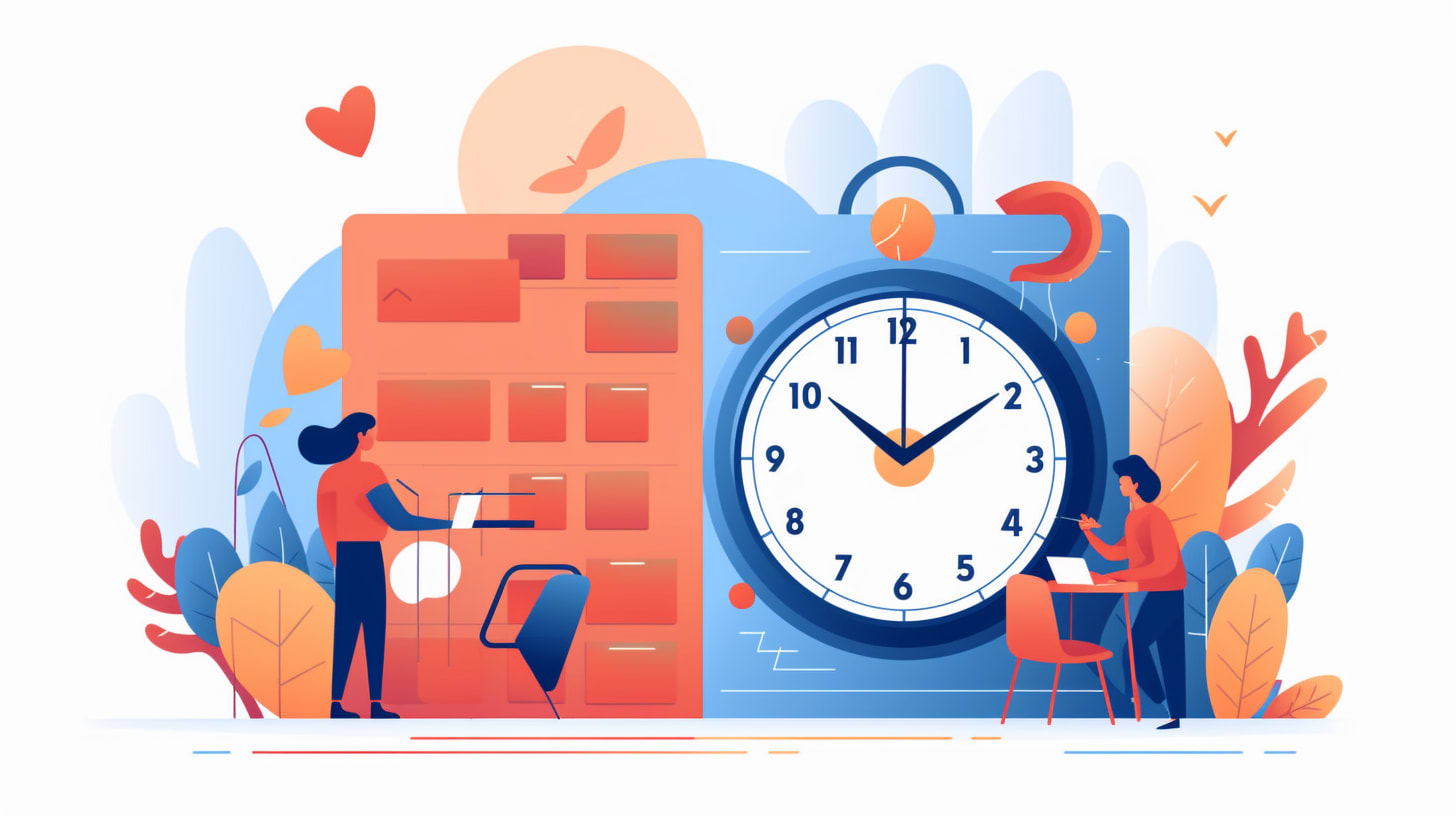,Scheduling AI assistants are tools that assist users in creating and automating the process of scheduling meetings and making appointments to helping others. Popular examples include Reclaim, Clockwise, Motion, Clara, Trevor, and Kronologic. These AI assistants offer various features to enhance efficiency and organization, such as automated scheduling, calendar integration, and task management.,For instance, Reclaim is a free AI calendar that also defines an ai assistant content seo with ai assistant that provides users with an ai powered editing streamlined experience, while clockwise is an AI scheduling tool with various features to optimize scheduling processes. Motion is an AI calendar that also functions as invest in quality ai project management app, and Clara is an AI scheduling faster with ai assistants may 2023 with 10web ai and content seo with ai assistant that helps users find a suitable time to meet someone.,By utilizing scheduling AI assistants, users can save time and effort in organizing their schedules and managing their own by setting reminders and making appointments beforehand.,Productivity AI assistants are AI-powered tools designed to facilitate the optimization of productivity by automating tasks and providing users with real-time insights and recommendations. Popular examples quality ai assistants include Google Assistant, Amazon Alexa, and Krisp, which all offer various features to enhance work efficiency.,Krisp, for instance, is an AI virtual assistant that eliminates background noise during calls and meetings, allowing users to maintain focus and productivity in noisy environments. Google Assistant and Amazon Alexa also provide features such as task automation, reminders, and organization tools to help users stay on top of their work.,By incorporating productivity AI virtual assistants to increase it, users can maximize their efficiency and effectiveness in managing their tasks and responsibilities.,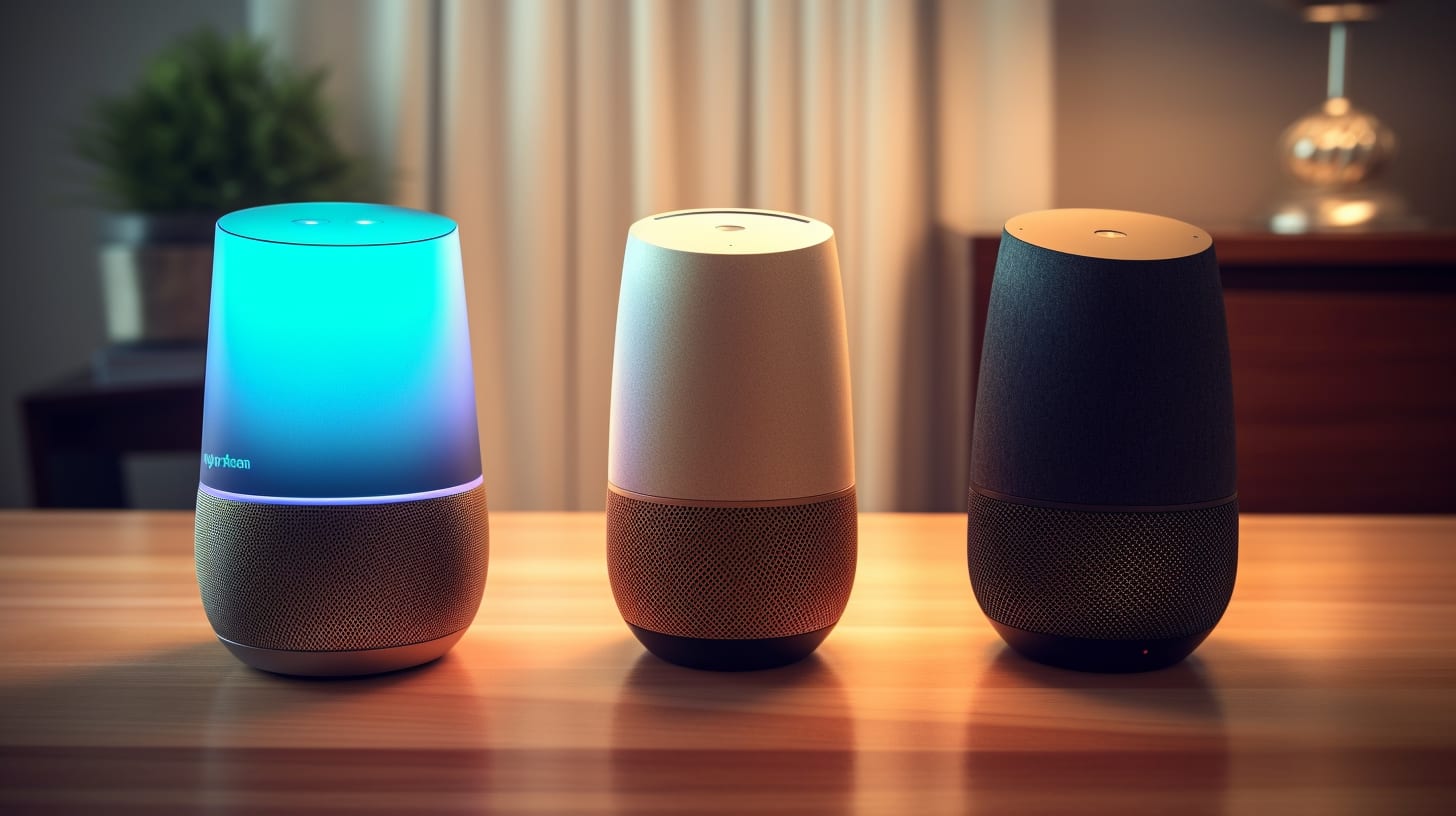,To summarize, the top AI assistants across categories include Amazon Alexa for personal assistant 6 best ai voice, top 25 ai assistants for virtual assistants, best ai writing assistants, Siri for voice assistant best, ios and android for android and desktop, 10 best ai assistants for personal, top 25 ai assistants for, AI Writing Assistant, Reclaim for scheduling assistant, and Krisp for productivity assistant.,Each of these AI assistants excels in its respective category, offering unique features and benefits to cater to users' diverse needs.,Amazon Alexa is the top personal AI assistant, offering a wide range of features such as creating to-do lists, setting alarms, playing audiobooks, streaming podcasts, and providing up-to-date information on news, weather, sports, and more. Alexa's compatibility with various devices and platforms makes it a popular choice for users seeking a versatile and feature-rich personal AI assistant.,With its user-friendly interface and extensive functionalities, Alexa has become a go-to choice for many individuals looking for a personal AI assistant that can help them stay organized, manage their schedules, and access information quickly and easily.,Siri is widely regarded as the top voice AI assistant, offering a plethora of features and capabilities that make it an excellent choice for users who prefer voice commands and interactions. Siri's advanced voice text and speech recognition, and natural language processing technology enable it to accurately understand and respond to user requests.,As a voice AI assistant, Siri provides services such as voice commands, voice searching, voice-activated device control, task handling, online information search, and real-time translation. Its compatibility with various Apple devices makes it a popular choice ai personal assistants among Apple users and those seeking a reliable and feature-rich voice AI assistant.,The 10Web AI Writing Assistant stands out best ai ai writing assistants, as the premier writing AI assistant, offering users valuable assistance in optimizing their content SEO by rectifying issues directly in Yoast, generating or rephrasing meta descriptions and titles, and correcting paragraph/sentence length errors. This AI assistant helps users produce high-quality, original content in a much shorter duration than manual writing would require, making it an invaluable tool for content creators.,By utilizing the AI Writing Assistant, users can expedite the process of producing high-quality content, create captivating and tailored content for their intended audience, optimize your content, and effectively optimize their content for search engines.,Reclaim stands out as the premier scheduling AI assistant, offering users a streamlined experience in organizing their schedules and managing their appointments. With many features and benefits such as automated scheduling, calendar integration, and task management, Reclaim is an invaluable tool for users looking to save time and effort in organizing their schedules.,By utilizing Reclaim, users can effectively manage their appointments and meetings, ensuring that they stay on top of their schedules and maintain a high level of productivity and organization.,Krisp is the most effective AI assistant for productivity, providing users with a tool that eliminates background noise during calls and meetings. This feature allows users to maintain focus and productivity in noisy environments, ensuring that they can effectively communicate and collaborate with their colleagues and clients.,By incorporating Krisp into their workflow, users can maximize their efficiency and effectiveness in managing their tasks and responsibilities, ultimately leading to improved productivity and success in their personal and professional lives.,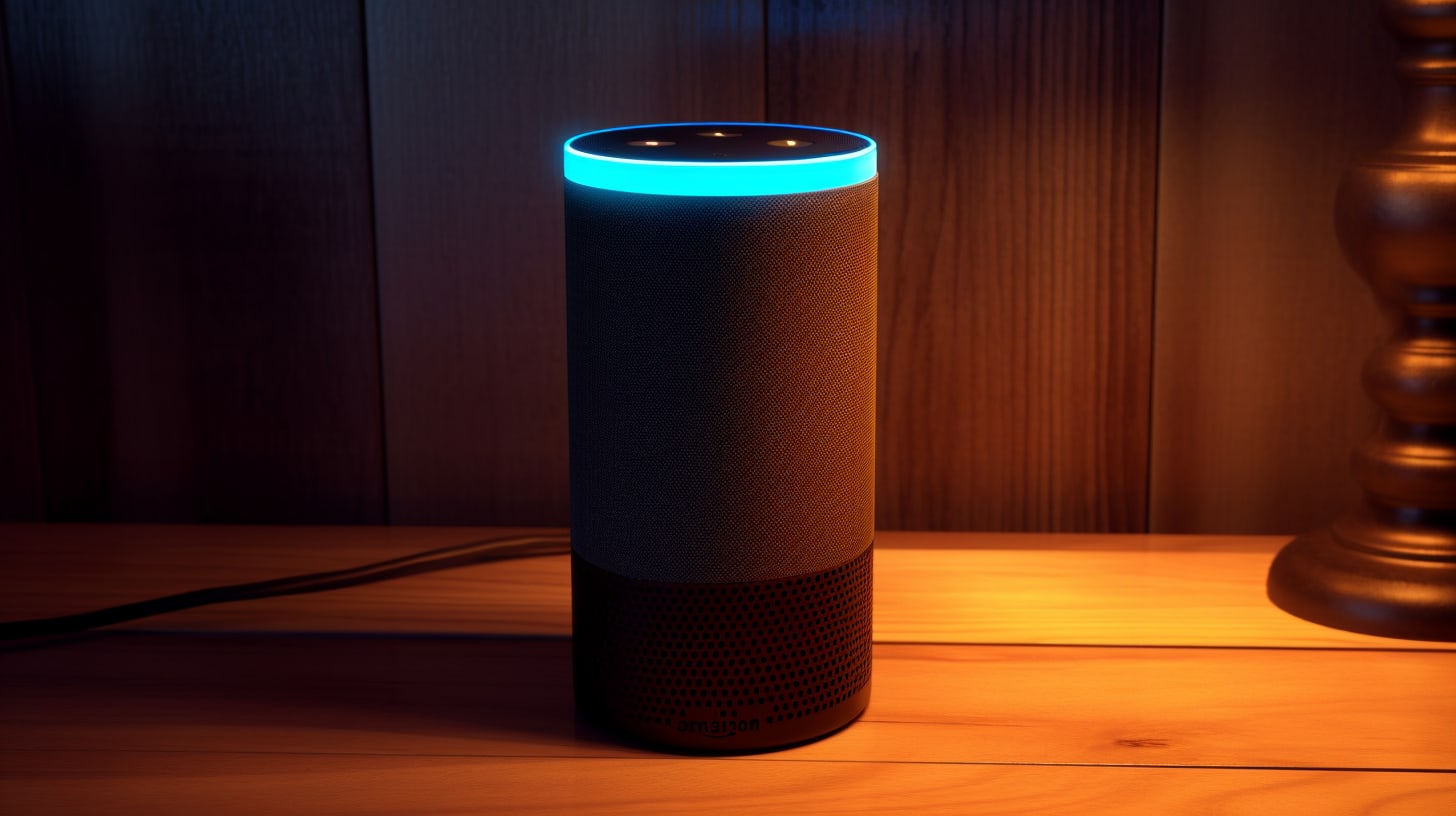,When comparing AI expectations from ai assistants around, it is important to consider factors such as features, pricing, user experience, and customer support. Understanding your needs and setting realistic your expectations for what 10 the top ai assistants have transformed 10 best ai assistants there may give you get from the 10 best ai assistants there may be is crucial in choosing the right AI assistant that best suits your requirements and preferences.,By researching and comparing various AI assistants based on these factors, you can make an informed decision and invest in a quality AI assistant that will effectively cater to your needs, enhance your productivity, and provide you with a seamless and enjoyable user experience.,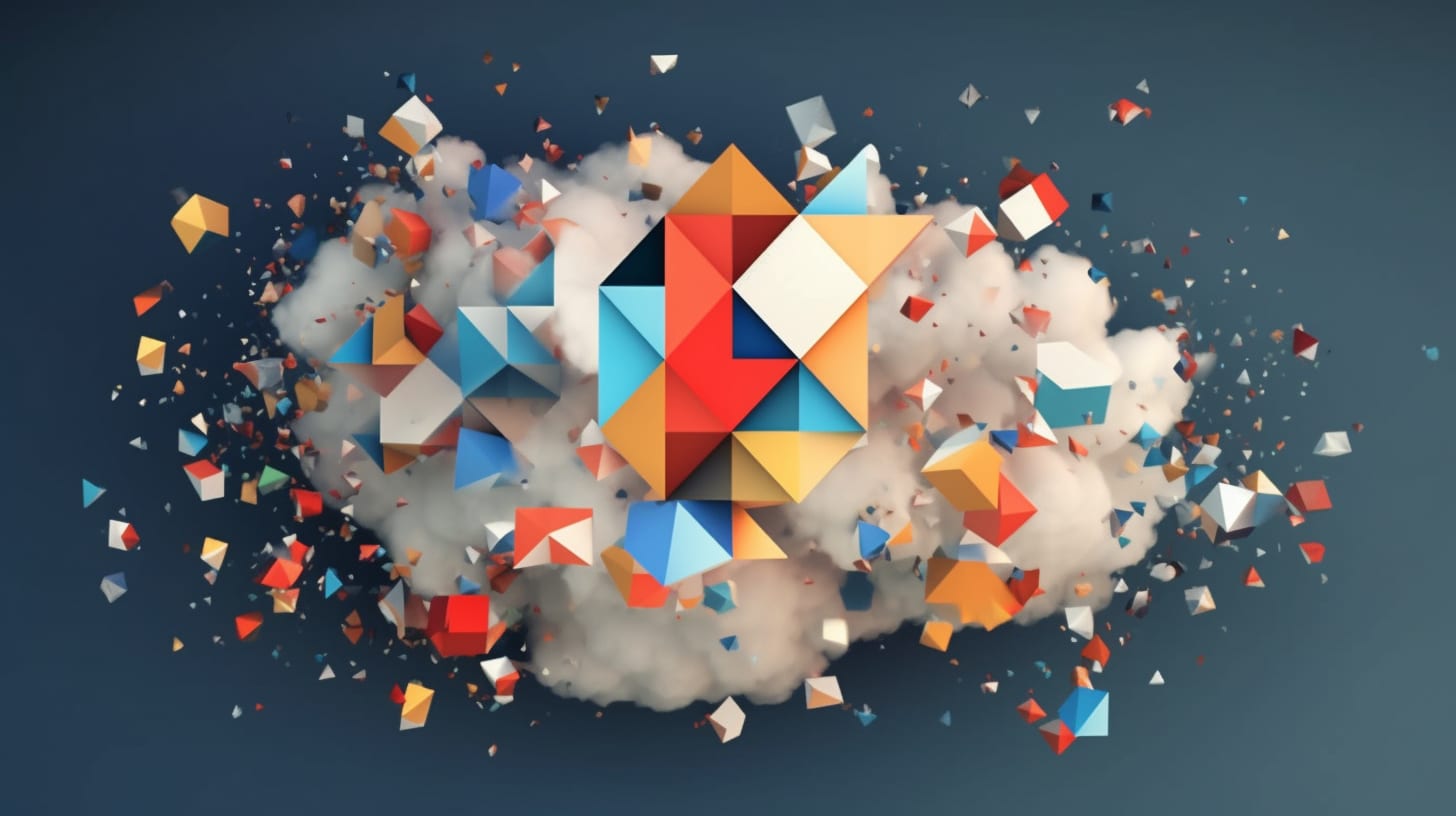,Privacy and security concerns with AI assistants include the amount of personal data they require to operate properly, such as location, contacts, and other individual details. Additionally, AI assistants are constantly listening, which may inadvertently capture voice exchanges and confidential information without the user's awareness. This can result in the unauthorized access of personal data and other sensitive information.,It is essential to be aware of these privacy and security concerns when using AI assistants and take necessary precautions to safeguard your personal information and maintain your privacy.,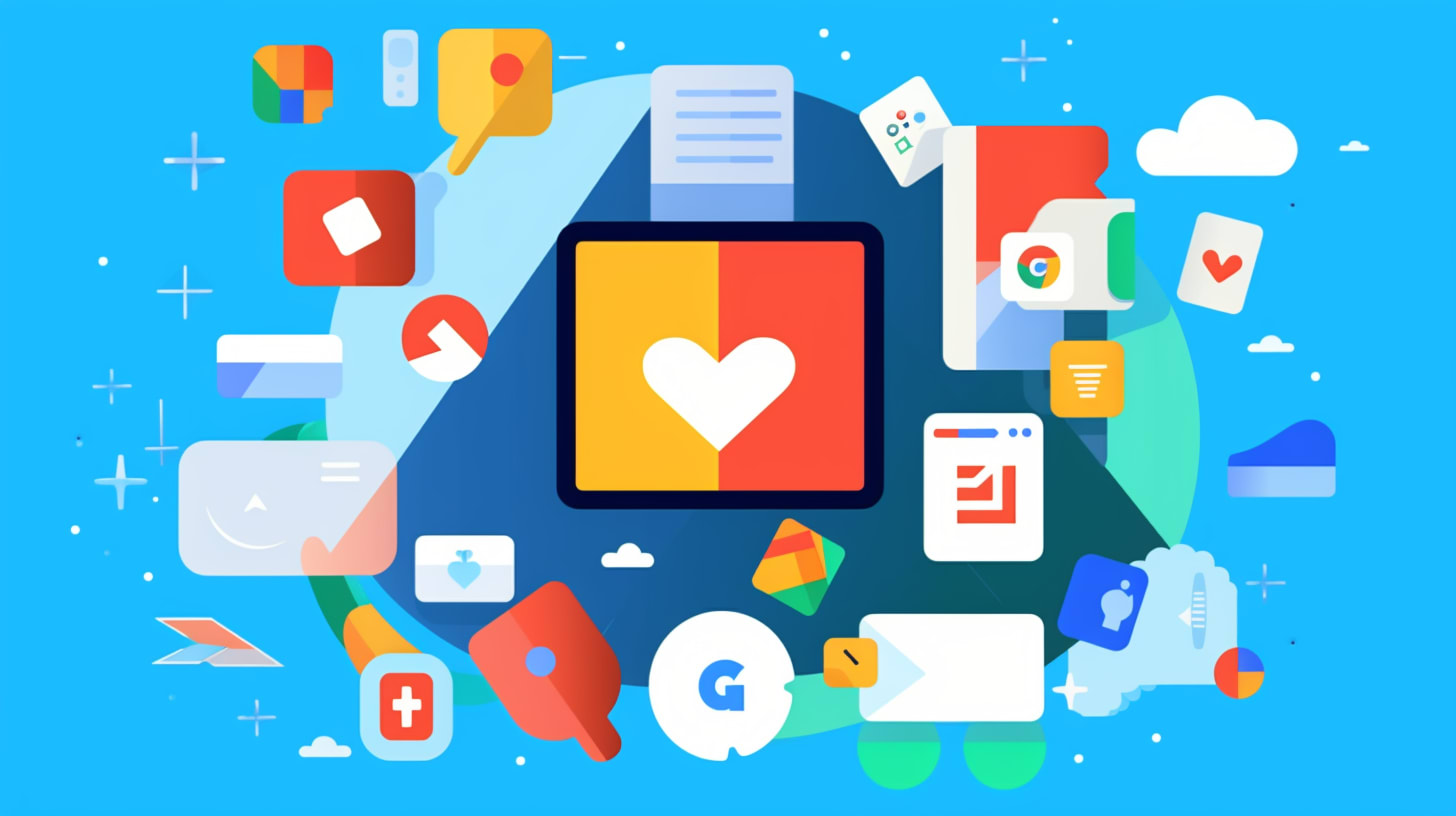,The future of AI quality ai assistants, is evolving and will soon be more humanistic, personalized, and ubiquitous. As AI technology progresses, it is anticipated that AI and ai virtual assistants, will become increasingly capable of delivering more individualized experiences. Gartner has predicted that by 2025, AI and ai virtual assistants, will become widespread, with approximately half of knowledge workers utilizing an ai powered app or selecting an ai assistant or ai powered virtual assistant on a regular basis.,With AI assistants becoming more sophisticated and versatile, it is essential to set realistic expectations and comprehend their capabilities to make the most of them. By choosing the appropriate AI assistant, users can enhance productivity, maintain organization, and accomplish objectives while taking advantage of its distinguishing features and advantages.,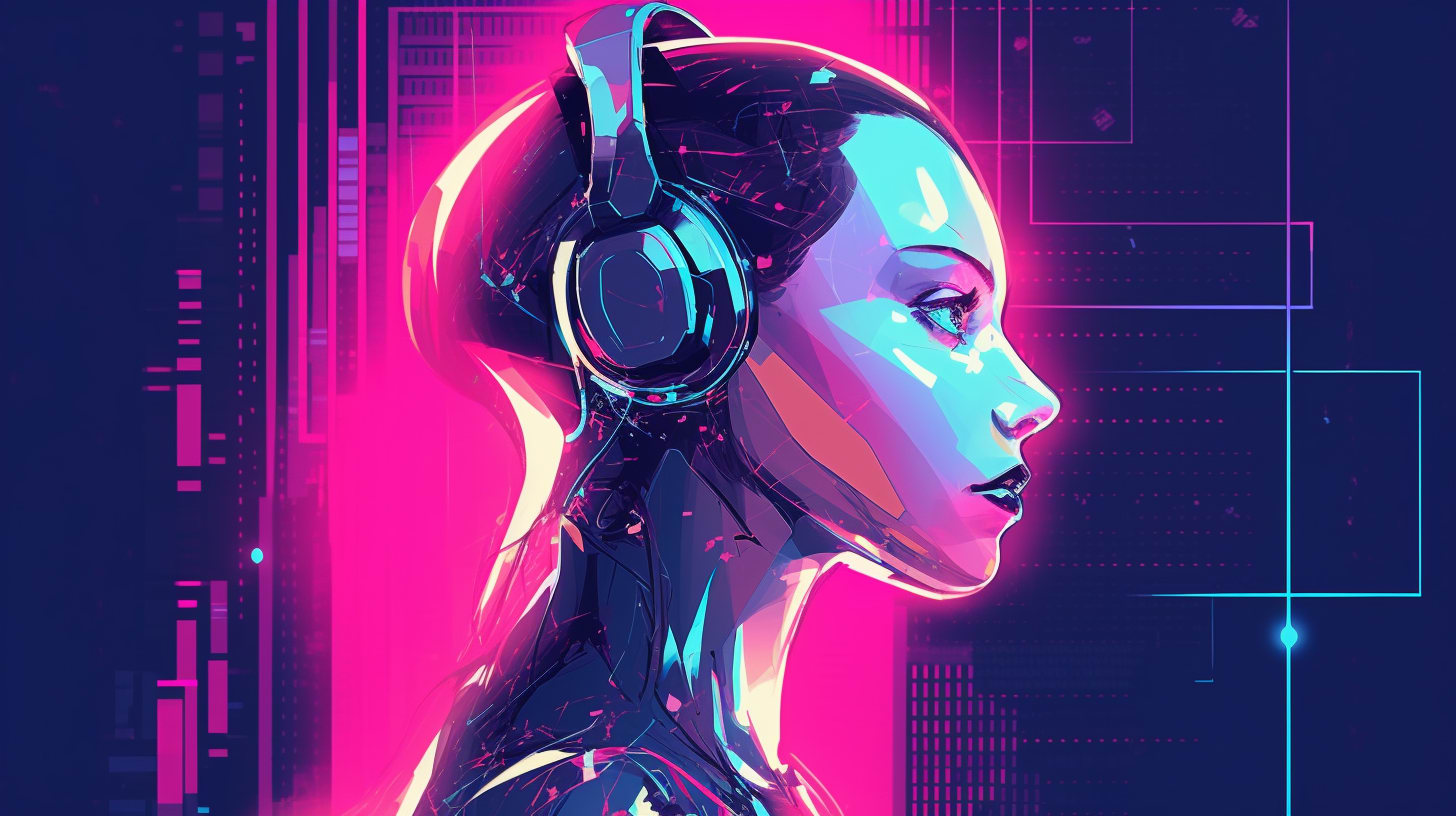,To choose the right AI assistant, it is crucial to first understand your needs and preferences best ai personal assistants. Determine what type of assistance you require and assess the AI assistant's compatibility with your devices and operating system. Evaluate the AI assistant's skillset and determine whether it is suitable for the tasks you require assistance with.,In addition, research features, pricing, user experience, and customer support when selecting an AI assistant. By considering these factors, you can make an informed decision and invest in a quality AI assistant, one that best suits your needs and all your needs and preferences.,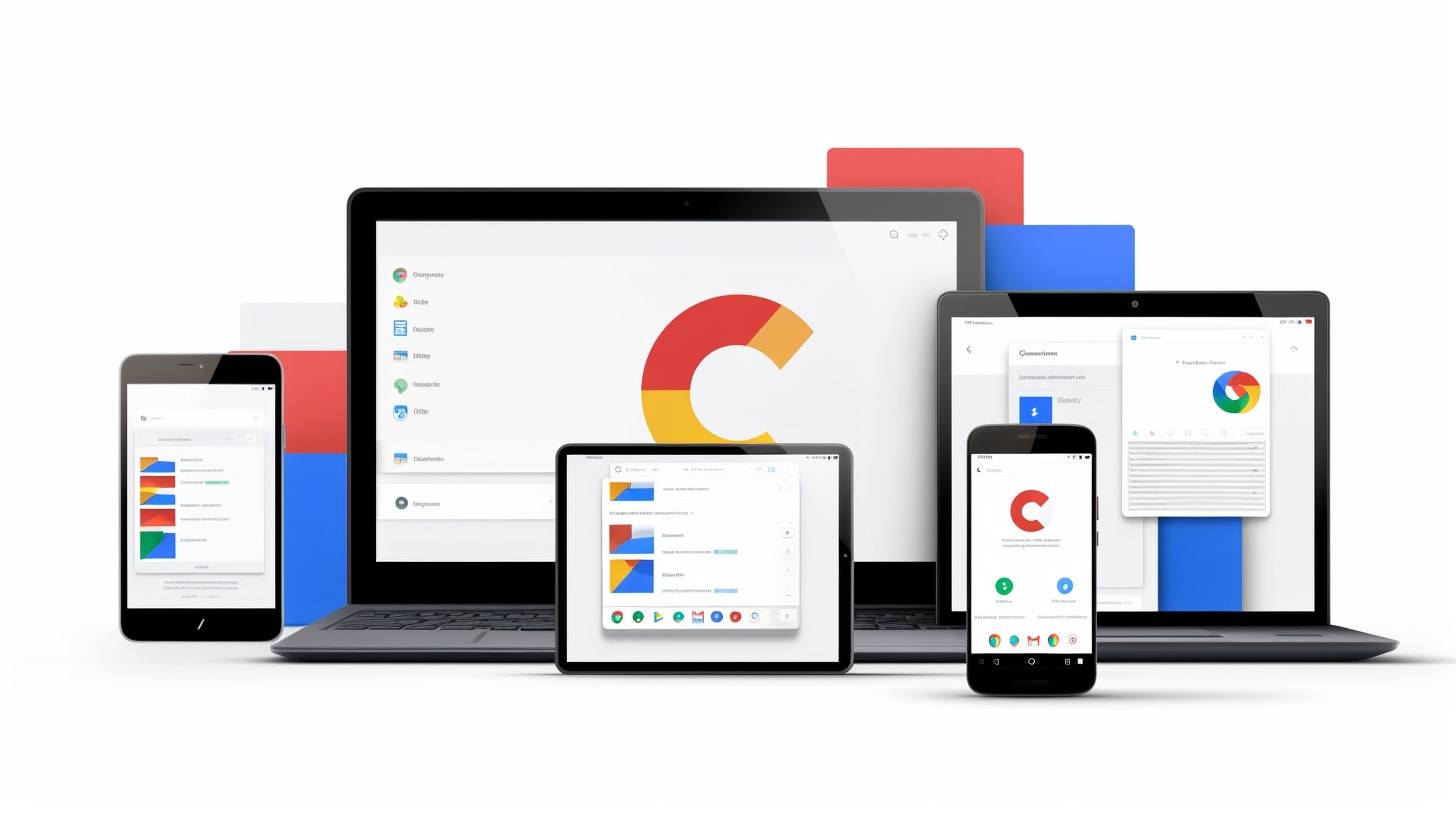,In conclusion, AI assistants have revolutionized the way we live and work, offering us unparalleled levels of assistance and support in various aspects of life. By understanding your needs, setting realistic expectations for what ai does, and researching features, pricing, user experience, and customer support, you can choose the right AI assistant that best suits your requirements and preferences. As AI technology continues to progress, we can expect AI assistants to become even more humanistic, personalized, and more ai assistants are becoming ubiquitous, further enhancing our productivity, helping us stay organized, and the appointments to helping us have success in both our personal and professional lives.